Open data and AI: A symbiotic relationship for progress
Unleashing the potential of this powerful duo
Artificial Intelligence (AI) systems are complex mathematical models trained on data. These systems are designed to process and analyse large volumes of data with the purpose of recognising patterns and making predictions. AI systems are becoming increasingly accurate and sophisticated due, in part, to advances in the techniques and algorithms used for AI, access to greater computer processing power, and the wider availability of data.
With these technological developments, the use of AI is becoming more common across sectors in Europe. Figure 1 shows how in 2021 the percentage of enterprises using AI technologies was varying across countries in Europe. To foster the use of AI across Europe, the EU launched the European AI Strategy aiming to boost research and industrial capacity while ensuring safety and fundamental rights.
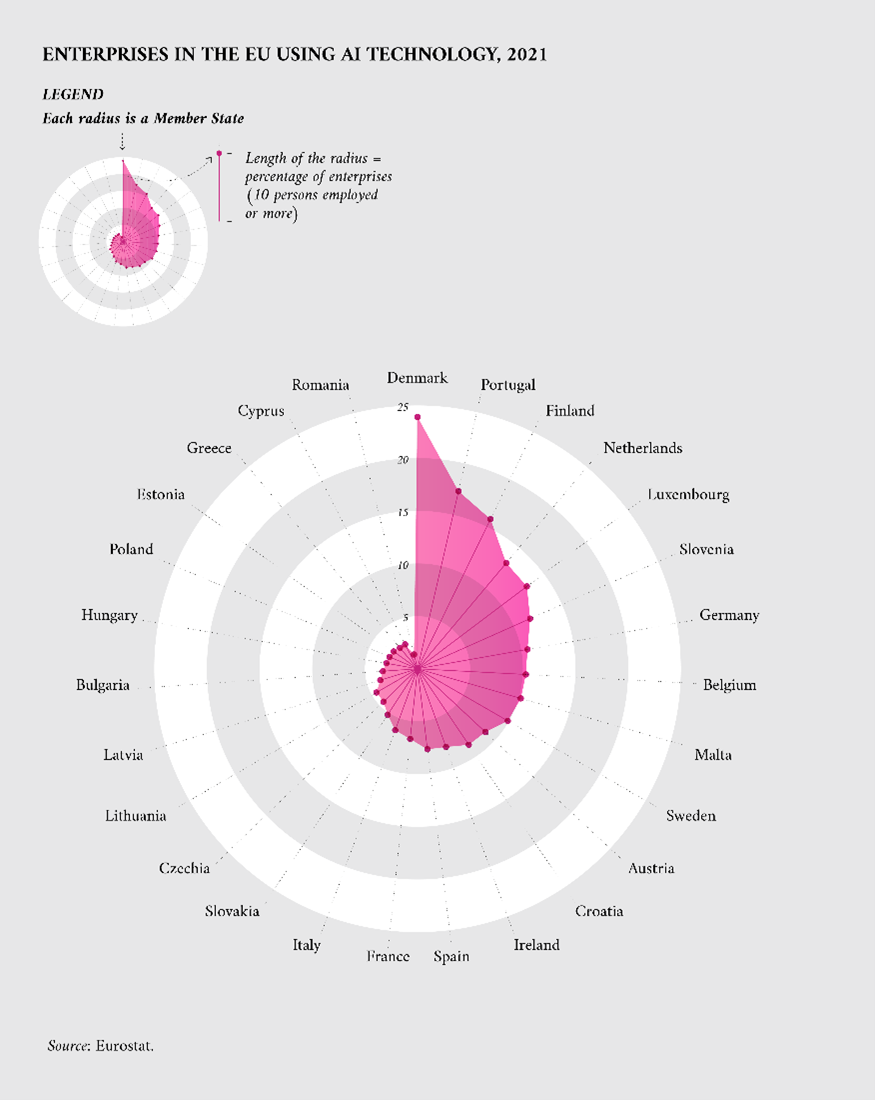
Figure 1: Percentage of enterprises in Europe using AI technology in 2021 (Eurostat)
Data is a critical component of AI systems. This story highlights the connection between open data and the performance of AI systems. With real-life use cases, this story showcases how these two topics are interlinked and are dependent on each other to foster Europe’s digital transition.
How open data and AI are closely related
Open data and AI have the potential to support and enhance each other’s capabilities. On the one hand, open data can improve AI systems. In general, exposing AI systems to a larger volume and variety of data increases the chance of the system returning accurate and useful predictions. As such, open data can be a supply of large amounts of diverse information for AI systems. In this way, the availability of open data contributes to better performing AI. For example, an AI system trained to predict consumer consumption patterns in Europe will likely perform better if it includes a representative selection of goods and service transactions by consumers across different countries, municipalities, and income groups.
On the other hand, AI can unlock additional value from open data. AI can analyse large volumes of data and identify trends and patterns that might not have been revealed through other analysis techniques. Open data contains rich information and complex patterns from which insights can be derived. As a powerful analysis tool, AI can thereby leverage the value of open data. For example, an AI system trained to predict forest fires can search for patterns across weather data, satellite images, and historical trends that standard statistical comparisons cannot identify.
AI can benefit from the breadth of open data
Open data encompasses a broad range of subject areas. This variety of data widens the possible use cases for which AI systems can be developed, making new AI-driven products and services possible. These use cases can only be developed if the relevant data is available and easy-to-access. The free availability of diverse datasets as through open data, is essential to drive innovation and bring new economic opportunities. The hope is that innovative AI systems can then be used to help solve challenges facing society, creating socioeconomic value.
The diverse pool of information that open data can provide for AI systems especially can enable multidisciplinary applications that combine data on several topics to derive new insights. For example, a weather dataset can be used to make weather predictions. But combined with data on seed genetics, soil characteristics, and environmental conditions, an AI system can be trained to have good contextual knowledge of the variables affecting agriculture production. Such a system could be used to aid decision-making to increase crop yields, prevent plant disease, or optimise other business decisions.
AI can benefit from the depth of open data
While the breadth of subject areas covered by open data enables broad use cases for AI systems, having extensive open data available on a specific use case allows AI systems to perform better. Take, for example, an AI system developed to recognise residential buildings. If the AI is trained on only images captured in the summertime of mansions in the countryside, the model will perform poorly when asked to recognise a city apartment as a residential building. The model therefore needs to be trained on a comprehensive set of examples to understand the variations of what is considered a residential building (in this case factors such as architectural style, building size, and surrounding environment may be relevant).
Data that comprehensively represent the subject matter exposes AI systems to a broader range of scenarios and variations. Ultimately, this allows AI models to perform better in real-world situations and generalise their knowledge when confronted with new data (such as a picture of an individual house that the model has not seen before). AI systems trained on unrepresentative or incomplete data risks making biased predictions and being unreliable.
High-quality AI relies on high-quality open data
The completeness of open data contributes to the ability of AI systems to generalise to unseen examples once these are deployed into “real-world” operation, but it also contributes to the concept of data quality. Some characteristics of data quality includes the completeness, relevance, consistency, uniformity, and trustworthiness of the data for the use case being developed. Figure 2 displays the relationship between six data quality dimensions and the performance of three types of AI algorithms from a paper by researchers at the University of Potsdam. For example, the figure shows that completeness (no missing data) and feature accuracy (no erroneous data) have a strong effect on the performance of all three AI algorithms. On the other hand, clustering algorithms are less affected by target accuracy (no mislabelling of data), uniqueness (no redundant or duplicated data), and class balance (having groups equally represented).
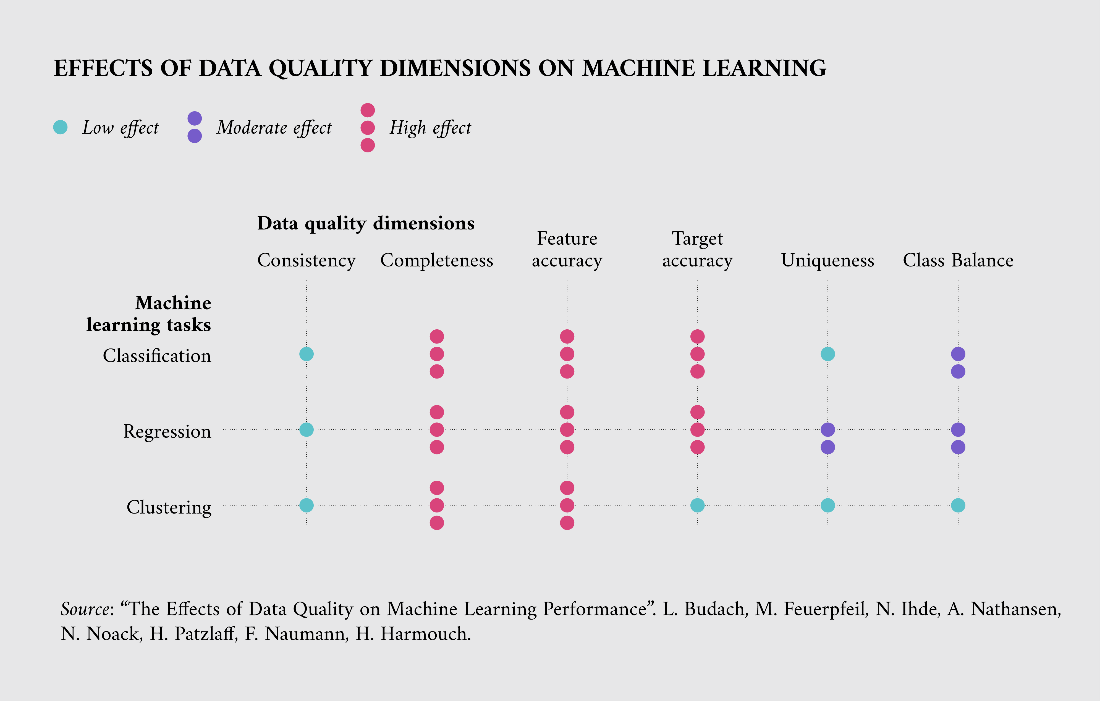
Figure 2: The effect of data quality on the performance of AI algorithms (GitHub)
Several open data initiatives in the scientific field demonstrate the impact of open repositories with structured catalogues of data and standardised data formats. For example, the German government funds a national research data infrastructure including a consortium (called NFDI4Chem) that makes chemical data findable, accessible, interoperable, and reusable by setting best practices including machine-readable chemical structures. These databases typically include a quality review process or curation method to ensure data quality and trustworthiness.
A greater demand for open data for new products and services might encourage the release of more datasets and improvements in data quality. It is therefore promising to see in the Open Data Maturity Report 2022 how European countries are working to improve the quality of the data published on their national open data portals.
Open data is enabling real-world AI applications
There are several examples of open data being used in AI systems for novel applications in Europe.
As a first example, the Croatian CROZ RenEUwable app combines climate and energy data into a machine learning model that provides citizens with personal recommendations for adopting more sustainable decisions on energy. The AI-driven app, which won the EU Datathon 2022 in the category of ‘A European Green Deal’, is based on open data selected by the team based on quality, completeness, consistency, timeliness, and usability.
Another example is a project for the Land Registry and Topography Administration in Luxembourg that illustrates the use of AI to analyse aerial images saved in geographic databases. Figure 3 displays an annual series of such aerial photographs. These databases need to be continuously updated and maintained, with new aerial images added every year. This task includes identifying all newly constructed, demolished, or updated buildings. Performing such an inspection manually is very laborious, so the Luxembourgish government launched a project to develop a proof of concept of an AI-based tool that can automatically identify changes to buildings. The project produced satisfying results and a goal for subsequent versions is to include other topographical objects such as walking paths.
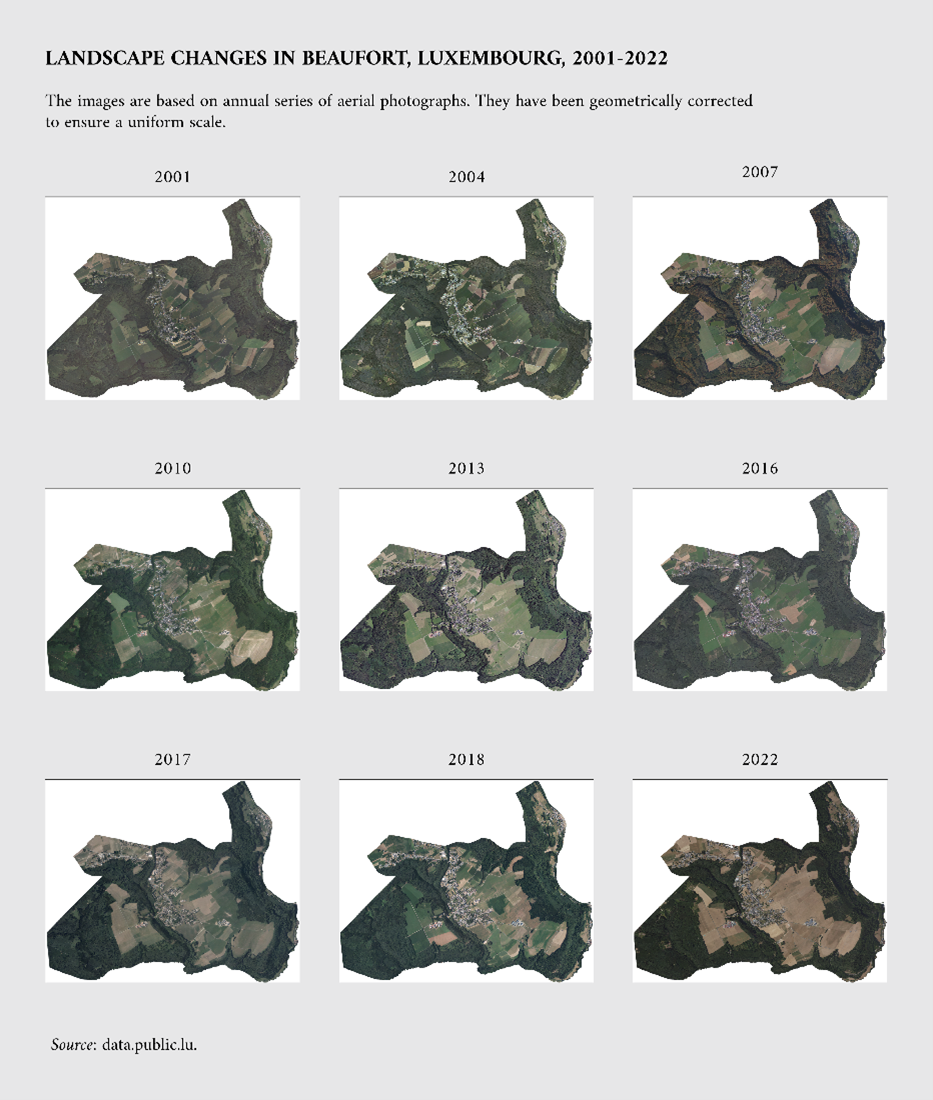
Figure 3: Landscape changes in Beaufort, Luxembourg from 2001 – 2022. Aerial images have been geometrically corrected to ensure a uniform scale (Ministry of Finance, Luxembourg)
As a final use case example, the European Commission launched the European Cancer Imaging Initiative to leverage data and digital technologies, such as AI, to combat cancer. This initiative aims to create an open dataset that links all existing resources and databases across Europe, working towards a more open, available, and user-friendly infrastructure for cancer imaging. The infrastructure is expected to be completed by December 2023, after which data providers will be able to connect to the platform.
Conclusion
The potential of AI systems in society is vast. When combined with open data, new opportunities become possible both for deriving new insights from open data and powering AI systems for new uses. The free availability of open data provided to all citizens without limits on its reuse enables enterprises to implement this data in their AI systems. Novel use cases are supported by the diversity of open data both in its potential breadth, which would offer multiple use cases for AI systems, and its potential depth, which would offer a deep representation of a specific use case. Policy initiatives that promote the sharing of certain datasets support the diversity of open data in Europe.
Increasing access to high-quality open data is a priority to unlock the synergy between open data and AI. Further improvements to data quality are supported by policies and community initiatives that impose quality standards and curation methods on open data. Data.europa.eu contributes to this goal through, among other means, its metadata quality dashboard which intends to help data providers and national data portals evaluate their metadata against various indicators such as accessibility and reusability.
If you want to find out more about the work of data.europa.eu and real examples on what to do with data, don´t miss out on the Use Case Observatory report and related learnings on the data.europa academy. Also, stay tuned for our next data stories and webinars by subscribing to our newsletter and following data.europa.eu on social media.